8 Reasons Why Data Programs Fail
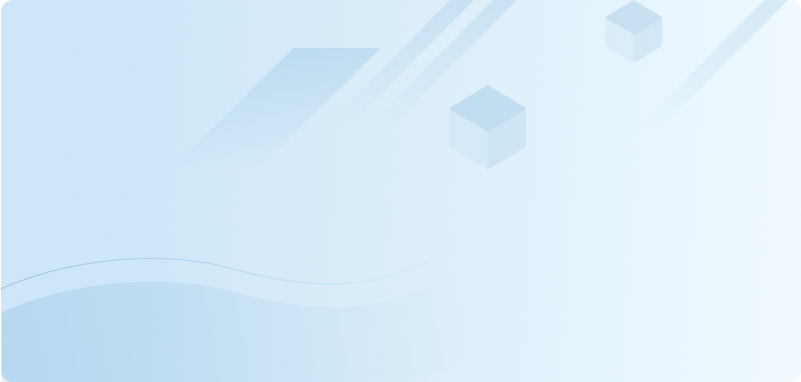
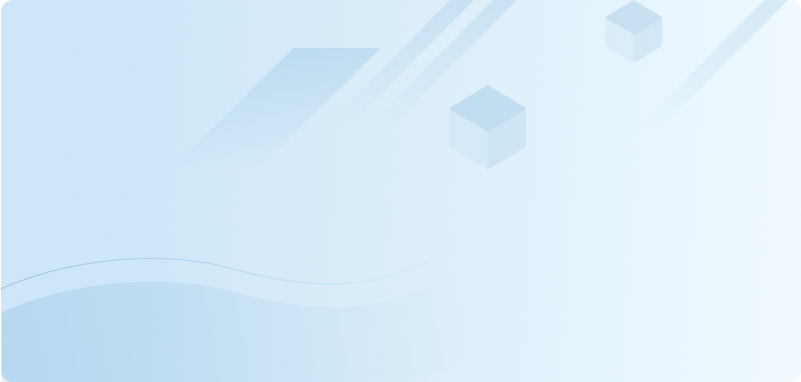
Why Do Data Programs Fail?
- Lack of clear goals and objectives: Without a clear understanding of what the data project is trying to achieve, it can be difficult to measure success and make decisions about how to move forward.
At North Labs, we call this our "North Star," it helps us answer the age-old question: "Why do we care?"
- Insufficient resources: Data projects require a significant investment of time, money, and personnel. If resources are not allocated appropriately, the project is likely to suffer.
You can't "kinda" build a successful data program, and you'll never hear a CIO say, "We just cobbled this thing together and are lucky it hasn't burned to the ground yet."
- Lack of buy-in from stakeholders: If key stakeholders do not support the data project, it can be difficult to secure the resources and support needed to see it through to completion.
Executives don't buy features and functionality--they buy stories and outcomes. They want a finishedhouse; they don't care about the brand of hammer you use. Figure out the North Star and make everymove align with that North Star.
- Complexity: Data projects can be highly complex, involving multiple systems and data sources, which can make them difficult to manage and increase the likelihood of errors.
Building a Proof of Concept is easy. Building a data program is so hard.
- Data quality issues: Poor quality data can lead to inaccurate or unreliable results, which can compromise the integrity of the entire project.
Your end product is only as reliable as your raw material. If your data inputs smell, your data output will smell worse.
- Insufficient testing: Data projects require thorough testing to ensure that they are functioning as intended and that any errors are identified and addressed before the project is launched.
Humans make (lots of) mistakes. Stop trying to eliminate the mistakes and build guardrails to detectand remediate the mistakes.
- Lack of flexibility: Data projects may need to be adapted as new information or requirements come to light. If a project team or environment is inflexible and unwilling to make changes, it can lead to project failure.
What you care about solving for today won't be anywhere near what you care about solving a year from now. Both your team and your environment need to be able to adapt over time.
- Inexperienced team: Data projects require specialized skills and knowledge. If the team members are inexperienced in data management, architecture, engineering, analytics, and visualization, it's more likely the project will fail.